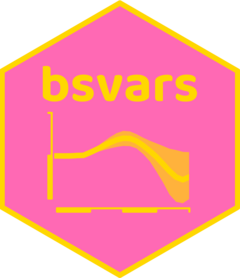
Provides posterior summary of heteroskedastic Structural VAR estimation
Source:R/summary.R
summary.PosteriorBSVARMSH.Rd
Provides posterior mean, standard deviations, as well as 5 and 95 percentiles of the parameters: the structural matrix \(B\), autoregressive parameters \(A\), and hyper parameters.
Usage
# S3 method for class 'PosteriorBSVARMSH'
summary(object, ...)
Arguments
- object
an object of class PosteriorBSVARMSH obtained using the
estimate()
function applied to heteroskedastic Bayesian Structural VAR model specification set by functionspecify_bsvar_msh$new()
containing draws from the posterior distribution of the parameters.- ...
additional arguments affecting the summary produced.
Value
A list reporting the posterior mean, standard deviations, as well as 5 and 95 percentiles of the parameters: the structural matrix \(B\), autoregressive parameters \(A\), and hyper-parameters.
Author
Tomasz Woźniak wozniak.tom@pm.me
Examples
# upload data
data(us_fiscal_lsuw)
# specify the model and set seed
set.seed(123)
specification = specify_bsvar_msh$new(us_fiscal_lsuw)
#> The identification is set to the default option of lower-triangular structural matrix.
# run the burn-in
burn_in = estimate(specification, 10)
#> **************************************************|
#> bsvars: Bayesian Structural Vector Autoregressions|
#> **************************************************|
#> Gibbs sampler for the SVAR-stationaryMSH model |
#> **************************************************|
#> Progress of the MCMC simulation for 10 draws
#> Every draw is saved via MCMC thinning
#> Press Esc to interrupt the computations
#> **************************************************|
# estimate the model
posterior = estimate(burn_in, 20)
#> **************************************************|
#> bsvars: Bayesian Structural Vector Autoregressions|
#> **************************************************|
#> Gibbs sampler for the SVAR-stationaryMSH model |
#> **************************************************|
#> Progress of the MCMC simulation for 20 draws
#> Every draw is saved via MCMC thinning
#> Press Esc to interrupt the computations
#> **************************************************|
summary(posterior)
#> **************************************************|
#> bsvars: Bayesian Structural Vector Autoregressions|
#> **************************************************|
#> Posterior summary of the parameters |
#> **************************************************|
#> $B
#> $B$equation1
#> mean sd 5% quantile 95% quantile
#> B[1,1] 0.1435034 0.005729775 0.13439 0.1520877
#>
#> $B$equation2
#> mean sd 5% quantile 95% quantile
#> B[2,1] -9.069875 0.5684475 -9.767726 -7.976045
#> B[2,2] 29.157633 1.8354469 25.596776 31.352969
#>
#> $B$equation3
#> mean sd 5% quantile 95% quantile
#> B[3,1] -24.810951 2.478346 -28.346178 -21.291206
#> B[3,2] -7.567592 1.319120 -9.433665 -5.784838
#> B[3,3] 41.081970 4.123952 35.445823 47.555374
#>
#>
#> $A
#> $A$equation1
#> mean sd 5% quantile 95% quantile
#> lag1_var1 1.198351472 0.04634731 1.14106229 1.25243864
#> lag1_var2 -0.006610039 0.01571539 -0.03459539 0.01138021
#> lag1_var3 -1.098950490 0.06192675 -1.16118306 -1.01773809
#> const 0.269570230 0.11701351 0.04831107 0.42794705
#>
#> $A$equation2
#> mean sd 5% quantile 95% quantile
#> lag1_var1 0.04884987 0.01235543 0.03275133 0.06712400
#> lag1_var2 0.96498340 0.01178477 0.94644942 0.98044309
#> lag1_var3 -0.31979441 0.01608034 -0.34358705 -0.29767062
#> const -0.20052801 0.09895587 -0.33938107 -0.06478152
#>
#> $A$equation3
#> mean sd 5% quantile 95% quantile
#> lag1_var1 0.154700318 0.03082854 0.10835293 0.19460779
#> lag1_var2 0.001491288 0.01074418 -0.01548196 0.01354322
#> lag1_var3 0.243082210 0.04110191 0.19476397 0.30806489
#> const 0.231658412 0.07822758 0.10629859 0.31613778
#>
#>
#> $hyper
#> $hyper$B
#> mean sd 5% quantile 95% quantile
#> B[1,]_shrinkage 106.6834 68.25505 20.74862 217.2337
#> B[2,]_shrinkage 261.3257 153.79204 84.38531 487.7074
#> B[3,]_shrinkage 294.7623 137.03565 113.99837 551.5747
#> B[1,]_shrinkage_scale 1011.5171 677.26941 232.66686 2220.7242
#> B[2,]_shrinkage_scale 1409.7907 980.51503 384.38202 2779.4770
#> B[3,]_shrinkage_scale 1269.7104 821.69210 425.40320 2646.5038
#> B_global_scale 111.0278 74.37812 37.37958 205.4944
#>
#> $hyper$A
#> mean sd 5% quantile 95% quantile
#> A[1,]_shrinkage 0.6855595 0.5153140 0.2436156 1.4773992
#> A[2,]_shrinkage 0.3768546 0.1803720 0.1624556 0.6499691
#> A[3,]_shrinkage 0.4271291 0.1827971 0.1686716 0.6804636
#> A[1,]_shrinkage_scale 6.0240787 2.4054085 3.2310428 9.2665831
#> A[2,]_shrinkage_scale 4.6029620 1.4117325 2.7351960 6.7531675
#> A[3,]_shrinkage_scale 5.2183527 2.3358892 2.4519483 8.7109091
#> A_global_scale 0.6223423 0.1501214 0.4425914 0.8776091
#>
#>
# workflow with the pipe |>
############################################################
set.seed(123)
us_fiscal_lsuw |>
specify_bsvar_msh$new() |>
estimate(S = 10) |>
estimate(S = 20) |>
summary()
#> The identification is set to the default option of lower-triangular structural matrix.
#> **************************************************|
#> bsvars: Bayesian Structural Vector Autoregressions|
#> **************************************************|
#> Gibbs sampler for the SVAR-stationaryMSH model |
#> **************************************************|
#> Progress of the MCMC simulation for 10 draws
#> Every draw is saved via MCMC thinning
#> Press Esc to interrupt the computations
#> **************************************************|
#> **************************************************|
#> bsvars: Bayesian Structural Vector Autoregressions|
#> **************************************************|
#> Gibbs sampler for the SVAR-stationaryMSH model |
#> **************************************************|
#> Progress of the MCMC simulation for 20 draws
#> Every draw is saved via MCMC thinning
#> Press Esc to interrupt the computations
#> **************************************************|
#> **************************************************|
#> bsvars: Bayesian Structural Vector Autoregressions|
#> **************************************************|
#> Posterior summary of the parameters |
#> **************************************************|
#> $B
#> $B$equation1
#> mean sd 5% quantile 95% quantile
#> B[1,1] 0.1435034 0.005729775 0.13439 0.1520877
#>
#> $B$equation2
#> mean sd 5% quantile 95% quantile
#> B[2,1] -9.069875 0.5684475 -9.767726 -7.976045
#> B[2,2] 29.157633 1.8354469 25.596776 31.352969
#>
#> $B$equation3
#> mean sd 5% quantile 95% quantile
#> B[3,1] -24.810951 2.478346 -28.346178 -21.291206
#> B[3,2] -7.567592 1.319120 -9.433665 -5.784838
#> B[3,3] 41.081970 4.123952 35.445823 47.555374
#>
#>
#> $A
#> $A$equation1
#> mean sd 5% quantile 95% quantile
#> lag1_var1 1.198351472 0.04634731 1.14106229 1.25243864
#> lag1_var2 -0.006610039 0.01571539 -0.03459539 0.01138021
#> lag1_var3 -1.098950490 0.06192675 -1.16118306 -1.01773809
#> const 0.269570230 0.11701351 0.04831107 0.42794705
#>
#> $A$equation2
#> mean sd 5% quantile 95% quantile
#> lag1_var1 0.04884987 0.01235543 0.03275133 0.06712400
#> lag1_var2 0.96498340 0.01178477 0.94644942 0.98044309
#> lag1_var3 -0.31979441 0.01608034 -0.34358705 -0.29767062
#> const -0.20052801 0.09895587 -0.33938107 -0.06478152
#>
#> $A$equation3
#> mean sd 5% quantile 95% quantile
#> lag1_var1 0.154700318 0.03082854 0.10835293 0.19460779
#> lag1_var2 0.001491288 0.01074418 -0.01548196 0.01354322
#> lag1_var3 0.243082210 0.04110191 0.19476397 0.30806489
#> const 0.231658412 0.07822758 0.10629859 0.31613778
#>
#>
#> $hyper
#> $hyper$B
#> mean sd 5% quantile 95% quantile
#> B[1,]_shrinkage 106.6834 68.25505 20.74862 217.2337
#> B[2,]_shrinkage 261.3257 153.79204 84.38531 487.7074
#> B[3,]_shrinkage 294.7623 137.03565 113.99837 551.5747
#> B[1,]_shrinkage_scale 1011.5171 677.26941 232.66686 2220.7242
#> B[2,]_shrinkage_scale 1409.7907 980.51503 384.38202 2779.4770
#> B[3,]_shrinkage_scale 1269.7104 821.69210 425.40320 2646.5038
#> B_global_scale 111.0278 74.37812 37.37958 205.4944
#>
#> $hyper$A
#> mean sd 5% quantile 95% quantile
#> A[1,]_shrinkage 0.6855595 0.5153140 0.2436156 1.4773992
#> A[2,]_shrinkage 0.3768546 0.1803720 0.1624556 0.6499691
#> A[3,]_shrinkage 0.4271291 0.1827971 0.1686716 0.6804636
#> A[1,]_shrinkage_scale 6.0240787 2.4054085 3.2310428 9.2665831
#> A[2,]_shrinkage_scale 4.6029620 1.4117325 2.7351960 6.7531675
#> A[3,]_shrinkage_scale 5.2183527 2.3358892 2.4519483 8.7109091
#> A_global_scale 0.6223423 0.1501214 0.4425914 0.8776091
#>
#>